
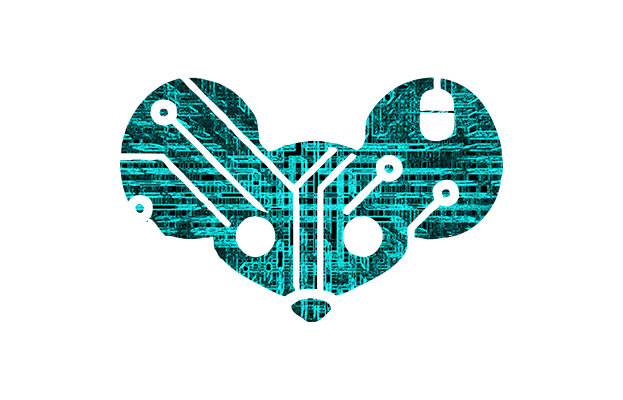
You can control that with a setting. In Settings - Privacy, turn on “Query in the page’s title.”
My instance has a magnifying glass as the favicon.
You can control that with a setting. In Settings - Privacy, turn on “Query in the page’s title.”
My instance has a magnifying glass as the favicon.
Giant squids are the bears of the ocean
Giphy has a documented API that you could use. There have been bulk downloaders, but I didn’t see any that had recent activity. However you still might be able to use one to model your own script after, like https://github.com/jcpsimmons/giphy-stacks
There were downloaders for Gfycat - gallery-dl supported it at one point - but it’s down now. However you might be able to find collections that other people downloaded and are now hosting. You could also use the Internet Archive - they have tools and APIs documented
There’s a Tenor mass downloader that uses the Tenor API and an API key that you provide.
Imgur has GIFs is supported by gallery-dl, so that’s an option.
Also, read over https://github.com/simon987/awesome-datahoarding - there may be something useful for you there.
In terms of hosting, it would depend on my user base and if I want users to be able to upload GIFs, too. If it was just my close friends, then Immich would probably be fine, but if we had people I didn’t know directly using it, I’d want a more refined solution.
There’s Gifable, which is pretty focused, but looks like it has a pretty small following. I haven’t used it myself to see how suitable it is. If you self-host it (or something else that uses S3), note that you can use MinIO or LocalStack for the S3 container rather than using AWS directly. I’m using MinIO as part of my stack now, though for a completely different app.
MediaCMS is another option. Less focused on GIFs but more actively developed, and intended to be used for this sort of purpose.
Wouldn’t be a huge change at this point. Israel has been using AI to determine targets for drone-delivered airstrikes for over a year now.
https://en.m.wikipedia.org/wiki/AI-assisted_targeting_in_the_Gaza_Strip gives a high level overview of Gospel and Lavender, and there are news articles in the references if you want to learn more.
This is at least being positioned better than the ways Lavender and Gospel were used, but I have no doubt that it will be used to commit atrocities as well.
For now, OpenAI’s models may help operators make sense of large amounts of incoming data to support faster human decision-making in high-pressure situations.
Yep, that was how they justified Gospel and Lavender, too - “a human presses the button” (even though they’re not doing anywhere near enough due diligence).
But it’s worth pointing out that the type of AI OpenAI is best known for comes from large language models (LLMs)—sometimes called large multimodal models—that are trained on massive datasets of text, images, and audio pulled from many different sources.
Yes, OpenAI is well known for this, but they’ve also created other types of AI models (e.g., Whisper). I suspect an LLM might be part of a solution they would build but that it would not be the full solution.
As it is, you only see new comments if you scroll past the post again (and your client has refreshed it) or if you open it directly. If your client hasn’t updated the comment count or if you refresh your feed and the post falls off, you’ll never see it anyway.
A “Watch” feature would solve this better. If you watch a post, you get aggregated notifications for edits and comments on the post. If you watch a comment, you get aggregated notifications for replies to it or any of its children.
By aggregated notifications, I mean that you’d get one notification that said “The post you watched has been edited; 5 new comments” rather than a notification for each new comment.
Then, in addition to exposing a “Watch” action on posts and comments, clients could also enable users to automatically hide posts that are watched, either by marking them as hidden or by hiding watched posts without updates.
If the latter approach were taken, notifications might not even be necessary - the post could just get added back into the user’s feed when changes were made. It would result in a similar experience to forums, where new activity in a topic would bump it to the front, but it would only impact the people who were watching it.
You can kinda get that behavior by sorting your feed by Active, but this could be used with other sorting methods.
Thanks for clarifying! I’ve heard nothing but praise for Kagi from its users so that’s what I was assuming, but Searxng has also been great so I wouldn’t have been too surprised if you’d compared them and found its results to be on par or better.
By the way, if you’re self hosting Searxng, you can use add your own index. Searxng supports YaCy, which is an actively developed, open source search index and crawler that can be operated standalone or as part of a decentralized (P2P) network. Here are the Searxng docs for that engine. I can’t speak to its quality as I still haven’t set it up, though.
there is a better open source meta search engines
I already use Searxng and have never used Kagi, but I’m curious why you say that Searxng is “better.” Are you saying that because the quality of the searches is better, because it’s open source and Kagi isn’t, or for some other reason?
the law has already made it clear you cannot copyright the output of an LLM.
That’s true in this context and often true generally, but it’s not completely true. The Copyright Office has made it clear that the use of AI tools has to be evaluated on a case-by-case basis, to determine if a work is the result of human creativity. Refer to https://www.copyright.gov/ai/ai_policy_guidance.pdf for more details.
For example, they state that the selection and arrangement of AI outputs may be sufficient for a work to be copyrightable. And that’s without doing any post-processing of the AI’s outputs.
They don’t talk about situations like this, but I suspect that, if given a prompt like “Rewrite this paragraph from third person to first person,” where the paragraph in question is copyrighted, the output would maintain the same copyright as the input (particularly if performed faithfully and without hallucinations). Such a revision could be made with non-LLM technology, after all.
You don’t find the Cheese Pervert creepy?
Do you only experience the 5-10 second buffering issue on mobile? If not, then you might be able to fix the issue by tuning your NextCloud instance - upping the memory limit, disabling debug mode and dropping log level back to warn if you ever changed it, enabling memory caching, etc…
Check out https://docs.nextcloud.com/server/latest/admin_manual/installation/server_tuning.html and https://docs.nextcloud.com/server/latest/admin_manual/installation/php_configuration.html#ini-values for docs on the above.
Your Passkeys have to be stored in something, but you don’t have to store them all in the same thing.
If you store them with Microsoft’s Windows Hello, Apple Keychain, or Google Password Manager, all of which are closed source, then you have to trust MS/Apple/Google. However, Keychain is end to end encrypted (according to Apple) and Windows Hello is currently not synced to the cloud, so if you trust those claims, you don’t need to trust that they won’t misuse your data. I don’t know if Google’s offering is end to end encrypted, but I wouldn’t trust it either way.
You can also store Passkeys in a password manager. Bitwarden is open source (though they did recently introduce a proprietary, source available SDK), as is KeepassXC. 1Password isn’t open source but can store Passkeys as well.
And finally, you can store Passkeys in a compatible security key, like the YubiKey 5 series keys, which can each store 100 Passkeys. This makes them basically immune to being stolen. Note that if your primary interest in Passkeys is in the phishing resistance (basically nearly perfect immunity to MitM attacks) then you can get that same benefit by using WebAuthn as a second factor. However, my experience has been that Passkey support is broader.
Revoking keys involves logging into the particular service and revoking them, just like changing your password. There isn’t a centralized way to do it as far as I’m aware. Each Passkey is only used for a single service, after all. However, in the same way that some password managers will offer to automatically change your passwords, they might develop a similar for passkeys.
Do any of the iOS or Android apps support passkeys? I looked into this a couple days ago and didn’t find any that did. (KeePassXC does.)
You have your link formatted backwards. It should be Vaultwarden, with the link in the parentheses.
a talking collar isn’t likely to help … if the cat is even willing to wear the thing at all.
“Realistically,” Quagliozzi says, “that collar would just be saying ‘get this fucking collar off me’ all the time.”
My first thought was that ending the taxes at land ownership was shortsighted - all capital should be taxed - but then I looked up Georgism on Wikipedia and saw that that was basically already in scope (mostly by “including title of ownership for natural resources and other contrived privileges (e.g., intellectual property)”).
You could’ve scrolled down to the bottom, clicked on “Links,” then clicked on the repo link
The repo has instructions to install a Snap or build from source. If you build from source, it looks like you should download an archive from the releases page rather than just pulling from master.
You probably just need Google One and Youtube Premium, which includes Youtube Music Premium.
Of course, if you don’t care about YouTube Premium, you could instead get a family subscription to a different music streaming service - Spotify, Tidal, and Apple Music are all leagues better than Youtube Music, in my opinion.
I don’t personally recommend Google for anything, to be clear.
You should also be able to just put two spaces
At the end of a line to insert a line break.
Open-Webui published a docker image that has a bundled Ollama that you can use, too: ghcr.io/open-webui/open-webui:cuda
. More info at https://docs.openwebui.com/getting-started/#installing-open-webui-with-bundled-ollama-support
Heads up for future reference: the video ID is the same between Youtube and Invidious, so you can just replace the invidious domain (
inv.nadeko.net
in this case) withyoutube.com
.